
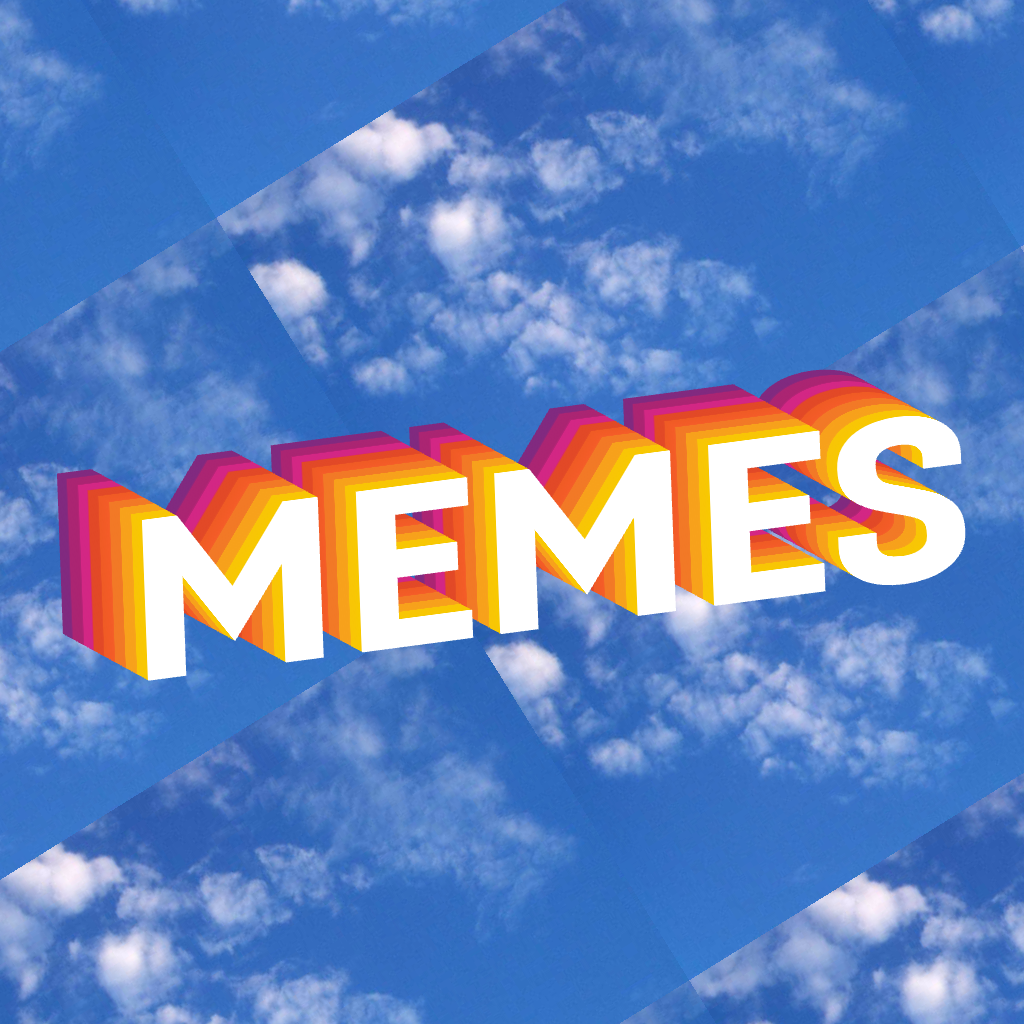
You may want to read through this as well as some case law on the subject before jumping to conclusions.
You may want to read through this as well as some case law on the subject before jumping to conclusions.
Not necessarily. You just need to demonstrate that you are running it like a for-profit company would, and with profit seeking motive.
Instead of a for-profit LLC, consider filing the business as a 501c charity.
Absolutely air traffic in the sky should be identified. There is no problem with that, but it’s the idea that it is too easy to find out everything about an aircraft owner by simply seeing the number on their tail.
The rich guys obfuscate that info with shell corps to own the aircraft.
Shouldn’t everyone have the right to the same level of privacy regardless of how much money they have?
No you cannot. You cannot easily find someone’s address from looking at their plate. You need more information, or to do some advanced searching. It is simply not the same.
It is different because you typically need to know the municipality I live in first.
Also the registration allows anyone to track me anytime I fly.
How would you feel if you had a public gps transponder on your car publicly showing who you, where you are, and where you live? Also what if you are required to plaster that registration number on the side of your vehicle in large letters that can be seen from a block away?
It’s a massive invasion of personal privacy.
Shitposters ride for free
This is actually most helpful to the little guys that own $20,000 airplanes.
I have a small airplane and it’s always bothered me that my name and address are publicly accessible through the FAA registry.
Most pilots I know are careful about photos they publish online showing their tail number printed in large bold letters on either side of the aircraft. This registration number can be entered into websites like flightaware.com and someone is literally two clicks from seeing my full name and home address.
Sell it to whom, Ben?
Well, OpenAI has clearly scraped everything that is scrap-able on the internet. Copyrights be damned. I haven’t actually used Deep seek very much to make a strong analysis, but I suspect Sam is just mad they got beat at their own game.
The real innovation that isn’t commonly talked about is the invention of Multihead Latent Attention (MLA), which is what drives the dramatic performance increases in both memory (59x) and computation (6x) efficiency. It’s an absolute game changer and I’m surprised OpenAI has released their own MLA model yet.
While on the subject of stealing data, I have been of the strong opinion that there is no such thing as copyright when it comes to training data. Humans learn by example and all works are derivative of those that came before, at least to some degree. This, if humans can’t be accused of using copyrighted text to learn how to write, then AI shouldn’t either. Just my hot take that I know is controversial outside of academic circles.
Yah, I’m an AI researcher and with the weights released for deep seek anybody can run an enterprise level AI assistant. To run the full model natively, it does require $100k in GPUs, but if one had that hardware it could easily be fine-tuned with something like LoRA for almost any application. Then that model can be distilled and quantized to run on gaming GPUs.
It’s really not that big of a barrier. Yes, $100k in hardware is, but from a non-profit entity perspective that is peanuts.
Also adding a vision encoder for images to deep seek would not be theoretically that difficult for the same reason. In fact, I’m working on research right now that finds GPT4o and o1 have similar vision capabilities, implying it’s the same first layer vision encoder and then textual chain of thought tokens are read by subsequent layers. (This is a very recent insight as of last week by my team, so if anyone can disprove that, I would be very interested to know!)
Maybe I want to move back into it… And selling has a 10% cost after realtor fees and closing fees.
Based on the amount of vitriol I’ve personally received on this site for renting one property while I am temporarily relocated to attend school, the answer is yes.
For some reason everyone views being a landlord as easy money. But in reality returns on investment are worse than the stock market for being the landlord of a single family home.
Ugh… This is all based on Dan Gryder’s YouTube channel. He’s not exactly the most trustworthy person. His channel is full of criticisms of pilots that have died flying that basically amount to “the pilot was dumb” and then when he causes a nearly mutli-fatal accident in a Lockheed 12A because he forgot to lock the tail wheel before landing, he feuds with the YouTuber that pointed this out.
He constantly derides the FAA and government in general, stating they are incompetent. He has stolen aircraft accident investigation evidence from a crash scene, a violation of federal statute. There’s the defamation lawsuit he lost against a pilot in 2023 to the tune of $1 million. And he was arrested in 2009 at an airport in Georgia.
Dan Gryder is an attention seeker who will say anything to receive publicity.
The Defense Production Act could be used to meet these ends. SpaceX is a defense contractor and exists at the privilege of the US Government for the US Government.
Coming from several people who work with SpaceX, there is a dedicated group of people that exist to distract Elon from all vital SpaceX functions.
SLS is on track to be more expensive when adjusted for inflation per moon mission than the Apollo program. It is wildly too expensive, and should be cancelled.
This coupled with the fact that the rocket is incapable of sending a manned capsule to low earth orbit which is the the lunar gateway is planned to a Rectilinear Halo Orbit instead.
Those working in the space industry know that SpaceX’s success is not because of Elon but instead Gwynne Shotwell. She is the President and CEO of SpaceX and responsible for all things SpaceX. The best outcome after the election is to remove Elon from the board and revoke his ownership of what is effectively a defense company for political interference in this election. Employees at SpaceX would be happy, the government would be happy, and the American people would be happy.
The technical definition of AI in academic settings is any system that can perform a task with relatively decent performance and do so on its own.
The field of AI is absolutely massive and includes super basic algorithms like Dijsktra’s Algorithm for finding the shortest path in a graph or network, even though a 100% optimal solution is NP-Complete, and does not yet have a solution that is solveable in polynomial time. Instead, AI algorithms use programmed heuristics to approximate optimal solutions, but it’s entirely possible that the path generated is in fact not optimal, which is why your GPS doesn’t always give you the guaranteed shortest path.
To help distinguish fields of research, we use extra qualifiers to narrow focus such as “classical AI” and “symbolic AI”. Even “Machine Learning” is too ambiguous, as it was originally a statistical process to finds trends in data or “statistical AI”. Ever used excel to find a line of best fit for a graph? That’s “machine learning”.
Albeit, “statistical AI” does accurately encompass all the AI systems people commonly think about like “neural AI” and “generative AI”. But without getting into more specific qualifiers, “Deep Learning” and “Transformers” are probably the best way to narrow down what most people think of when they here AI today.
This is truly a terrible accident. Given the flight tracking data and the cold, winter weather at the time, structural icing is likely to have caused the crash.
Ice will increase an aircraft’s stall speed, and especially when an aircraft is flown with autopilot on in icing conditions, the autopilot pitch trim can end up being set to the limits of the aircraft without the pilots ever knowing.
Eventually the icing situation becomes so severe that the stall speed of the ice-laden wing and elevator exceeds the current cruising speed and results in a aerodynamic stall, which if not immediately corrected with the right control inputs will develop into a spin.
The spin shown in several videos is a terrifying flat spin. Flat spins develop from normal spins after just a few rotations. It’s very sad and unfortunate that we can hear that both engines are giving power while the plane is in a flat spin towards the ground. The first thing to do when a spin is encountered is to eliminate all sources of power as this will aggravate a spin into a flat spin.
Once a flat spin is encountered, recovery from that condition is not guaranteed, especially in multi-engine aircraft where the outboard engines create a lot of rotational inertia.
Someone did the math and realized we would need a 130% tariff on all goods to replace current income tax revenue.
People’s number one concern is inflation. If that tariff is created we will see 100% inflation over night!
An LLC is a business. There’s no other way around it. The IRS will revoke your LLC if you are not running it as a business or under protected non-profit clauses.
Don’t take my word for it. Please consult with someone who has owned LLCs or even sole proprietorships for more than 5 years before charging ahead.
I’ve been running either an LLC or a sole proprietorship for 7 years, but I’m just random random internet person.
Also 1/3 of tax law are the actual words of any given law. The other 2/3 of tax law is executive interpretation/enforcement and case law from around the country.
There are some really interesting cases, even where tax lawyer firms get it wrong. In one instance a law firm tried to deduct their daily lunches as business meetings, and the tax court said no, even though it clearly states in the text of law that this is permissable. The judge basically said you can’t declare a daily lunch as a business meeting.
Other court documents can be found here:
https://www.taxnotes.com/research/federal/court-documents